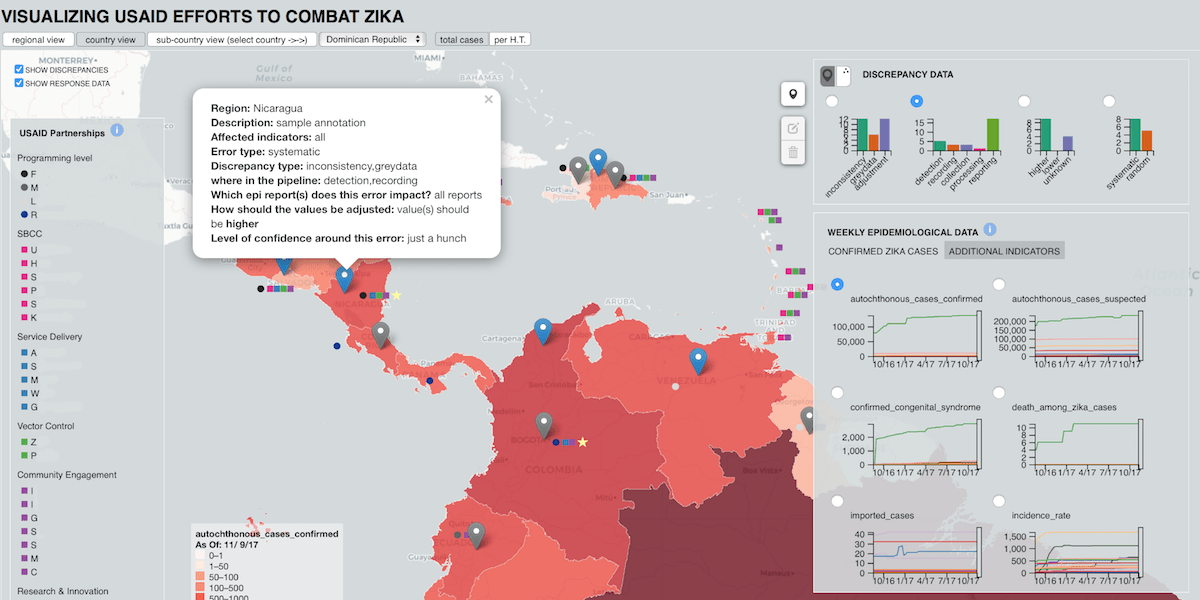
Abstract
This paper presents a framework for externalizing and analyzing expert knowledge about discrepancies in data through the use of visualization. Grounded in an 18-month design study with global health experts, the framework formalizes the notion of data discrepancies as implicit error, both in global health data and more broadly. We use the term implicit error to describe measurement error that is inherent to and pervasive throughout a dataset, but that isn’t explicitly accounted for or defined. Instead, implicit error exists in the minds of experts, is mainly qualitative, and is accounted for subjectively during expert interpretation of the data. Externalizing knowledge surrounding implicit error can assist in synchronizing, validating, and enhancing interpretation, and can inform error analysis and mitigation. The framework consists of a description of implicit error components that are important for downstream analysis, along with a process model for externalizing and analyzing implicit error using visualization. As a second contribution, we provide a rich, reflective, and verifiable description of our research process as an exemplar summary toward the ongoing inquiry into ways of increasing the validity and transferability of design study research.Citation
Nina McCurdy,
Julie Gerdes,
Miriah Meyer
A Framework for Externalizing Implicit Error Using Visualization
IEEE Transactions on Visualization and Computer Graphics (InfoVis), 25(1): 925-935, doi:10.1109/TVCG.2018.2864913, 2019.
BibTeX
@article{2018_infovis_ie-framework, title = {A Framework for Externalizing Implicit Error Using Visualization}, author = {Nina McCurdy and Julie Gerdes and Miriah Meyer}, journal = {IEEE Transactions on Visualization and Computer Graphics (InfoVis)}, publisher = {IEEE}, doi = {10.1109/TVCG.2018.2864913}, volume = {25}, number = {1}, pages = {925-935}, year = {2019} }
Acknowledgements
We thank our colleagues and collaborators for invaluable discussions regarding this work: Jason Dykes, City, College of London; Mike Kirby, University of Utah; and members of the Visualization Design Lab at the University of Utah. We also thank our collaborators at USAID. This work is supported in part by NSF grant GRF-1747505. The authors’ views expressed in this publication do not necessarily reflect the views of the United States Agency for International Development or the United States Government.