About
We are a team of visualization researchers at the University of Utah. Our interests include the process of designing and developing visualizations, visualization for biology, visualization frameworks, and, more generally, visualization of big, heterogeneous, and complex datasets.
VDL is part of the Scientific Computing and Imaging Institute and the School of Computing.
Blog And News
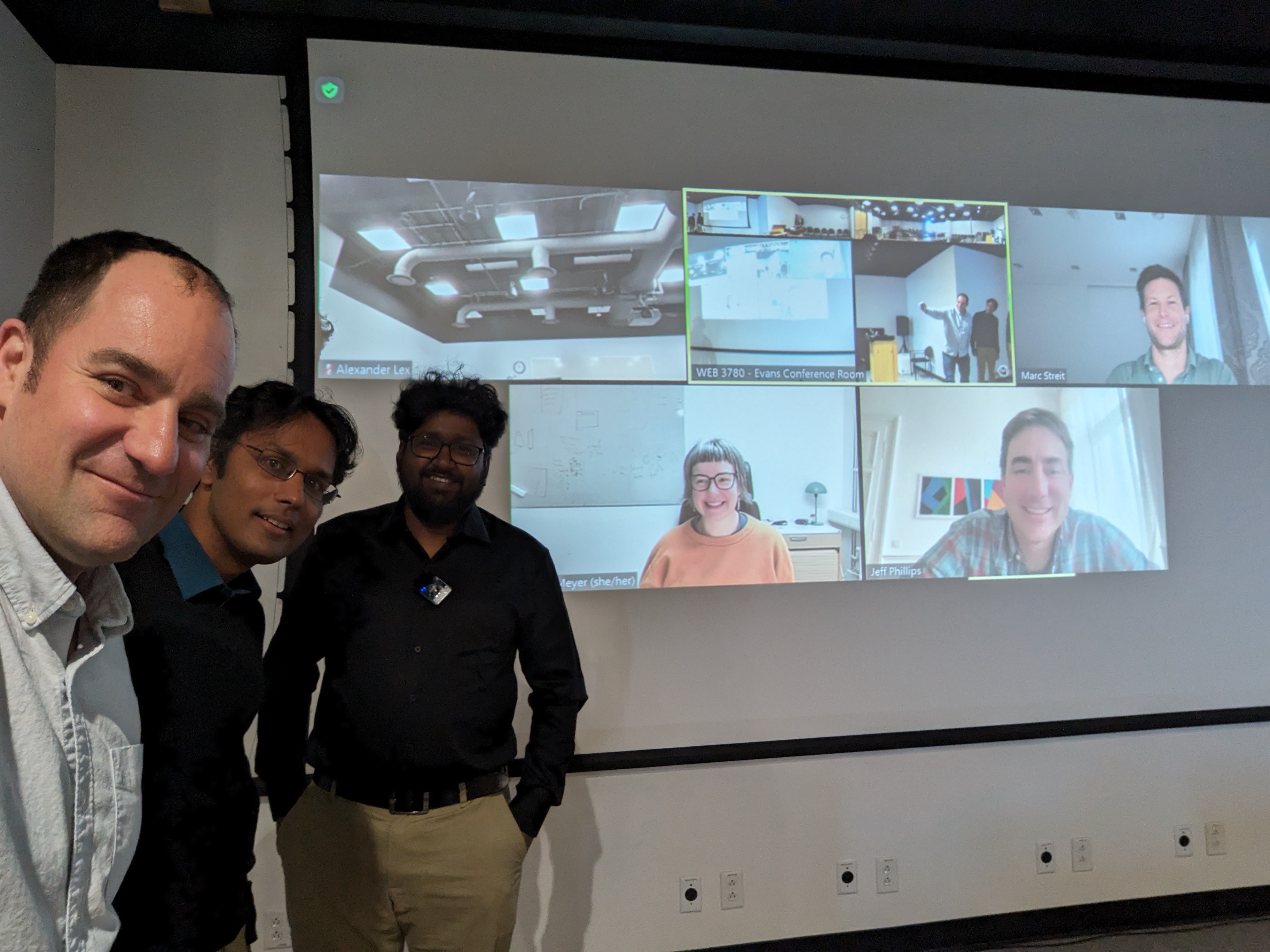
Kiran Gadhave successfully defended his dissertation on “Towards Reproducible and Reusable Visual Analysis”. Kiran was advised by Alex Lex, with Miriah Meyer, Jeff Phillips, Vivek Srikumar, and Marc Streit serving on the committee.
Congrats, and good luck with your next steps!
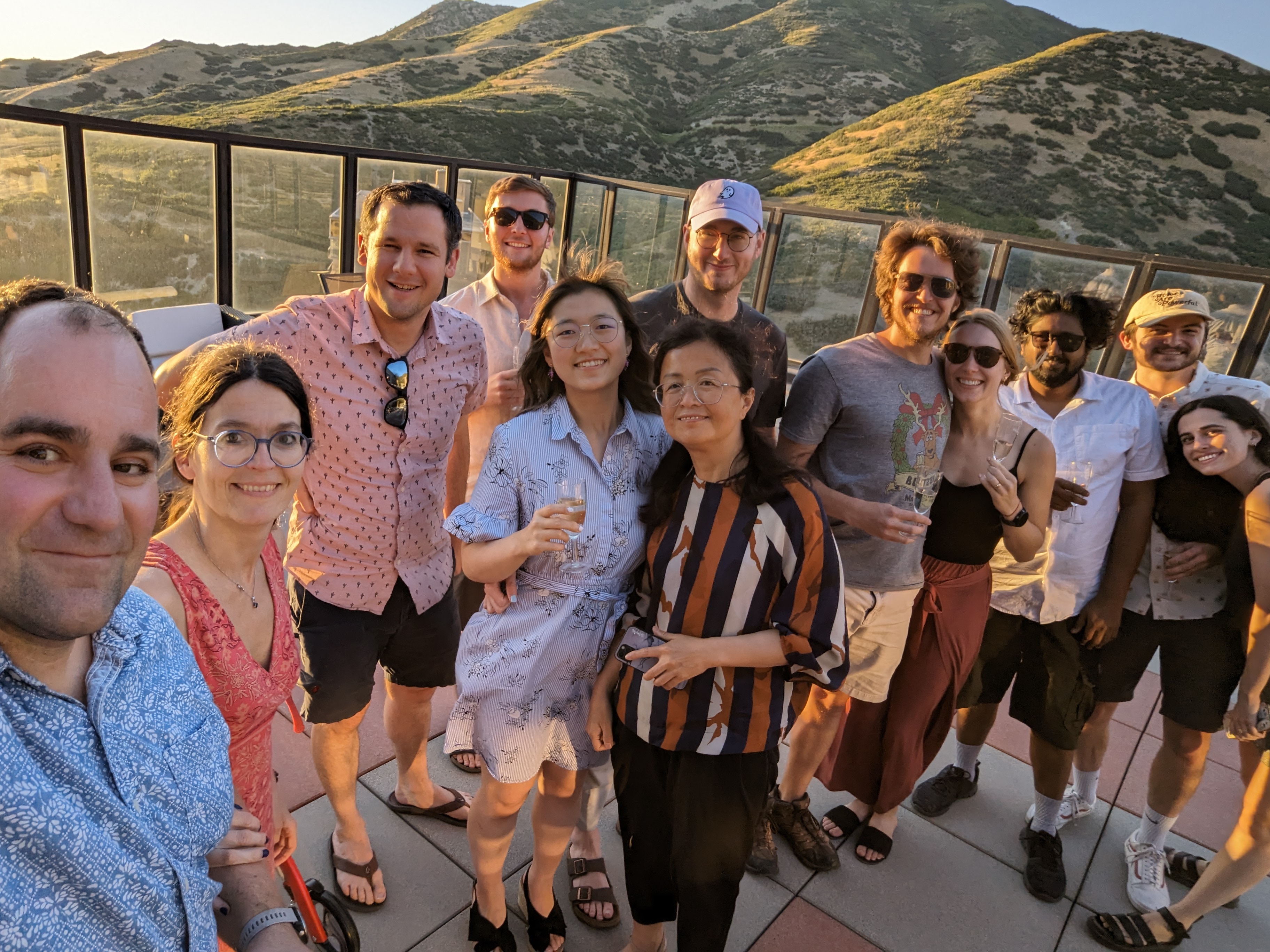
Haihan successfully defended her dissertation on “Data Hunches: Expressing Personal Knowledge in Data Visualizations”. Haihan was advised by Alex Lex, with Miriah Meyer, Marina Kogan, Jason Wiese, and Eytan Adar serving on the committee.
Haihan is staying in Utah and will join other VDL Alumni at Lucid Software. Congrats, and good luck with your next steps!
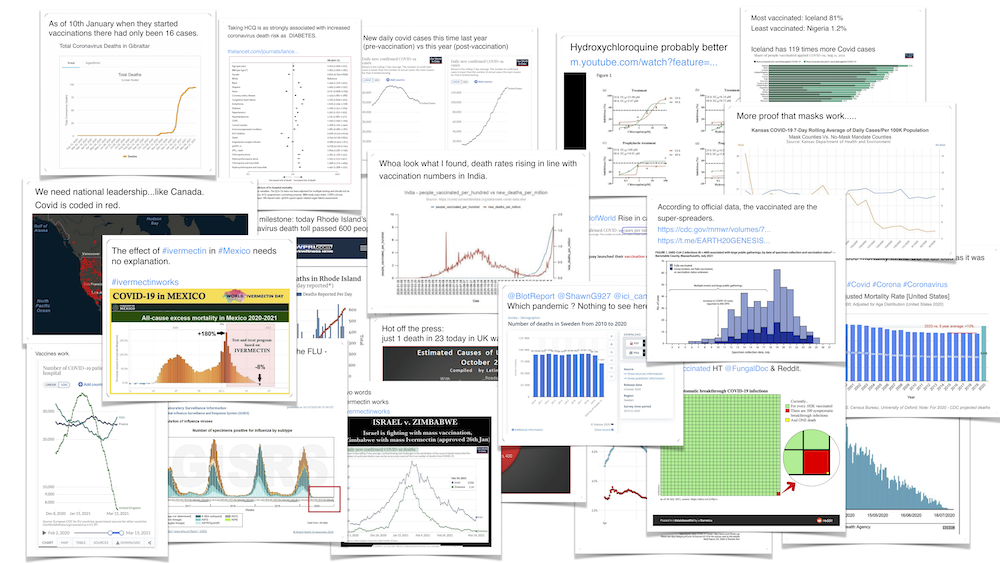