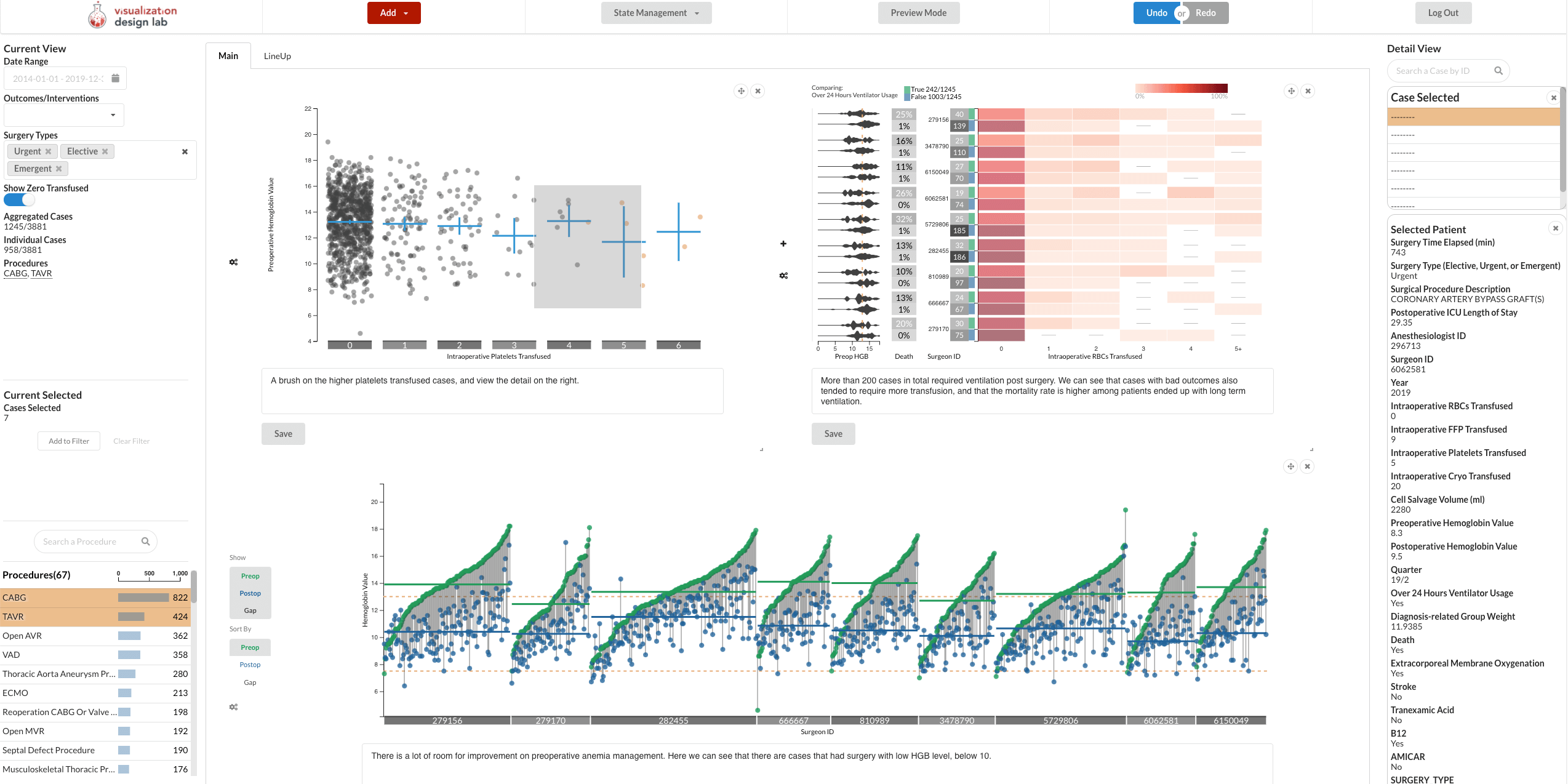
Abstract
Blood transfusion is a frequently performed medical procedure in surgical and nonsurgical contexts. Although it is frequently necessary or even life-saving, it has been identified as one of the most overused procedures in hospitals. Unnecessary transfusions not only waste resources but can also be detrimental to patient outcomes. Patient blood management (PBM) is the clinical practice of optimizing transfusions and associated outcomes. In this paper, we introduce Sanguine, a visual analysis tool for transfusion data and related patient medical records. Sanguine was designed with two user groups in mind: PBM experts and clinicians who conduct transfusions. PBM experts use Sanguine to explore and analyze transfusion practices and its associated medical outcomes. They can compare individual surgeons, or compare outcomes or time periods, such as before and after an intervention regarding transfusion practices. PBM experts then curate and annotate views for communication with clinicians, with the goal of improving their transfusion practices. Such a review session could be in person or through a shared link. We validate the utility and effectiveness of Sanguine through case studies.Citation
Haihan Lin,
Ryan A. Metcalf,
Jack Wilburn,
Alexander Lex
Sanguine: Visual Analysis for Patient Blood Management
Workshop on Visual Analytics in Healthcare at AMIA (VAHC), 2020.
BibTeX
@inproceedings{2020_vahc_sanguine, title = {Sanguine: Visual Analysis for Patient Blood Management}, author = {Haihan Lin and Ryan A. Metcalf and Jack Wilburn and Alexander Lex}, booktitle = {Workshop on Visual Analytics in Healthcare at AMIA (VAHC)}, year = {2020} }
Note
This is a workshop paper which was extended to a journal paper. Please refer to and cite the journal paper instead.
Acknowledgements
We thank Dr. Vikas Sharma, the Enterprise Data Warehouse, and the Center for High Performance Computing at the University of Utah. We gratefully acknowledge funding for this project by ARUP Laboratories. Computational resources used were partially funded by the NIH Shared Instrumentation Grant 1S10OD021644-01A1.